Applications of Quantum Machine Learning in Finance
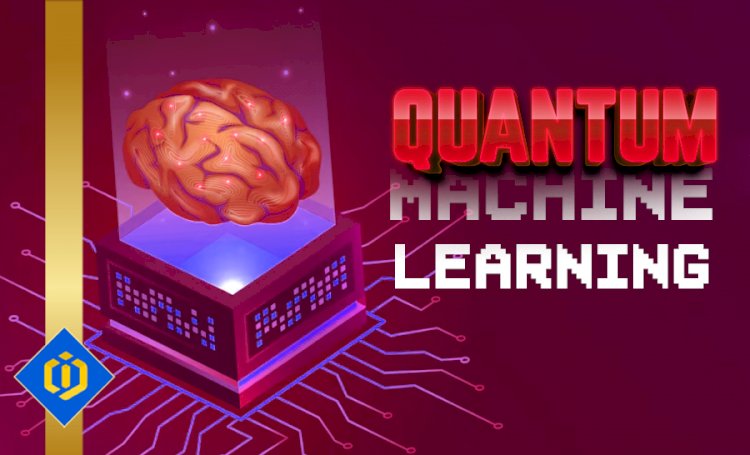
Even though there is a lot of potential for quantum computing to revolutionize the financial industry, there are still many obstacles to overcome. The following are a few of the major difficulties and restrictions.
Cost: Quantum hardware is currently much more expensive than classical hardware, making it difficult for smaller investors to adopt quantum computing methods. The specialized abilities and knowledge needed to operate quantum hardware can also be expensive.
Quantum algorithms are frequently much more complicated than classical algorithms, which can make it challenging to interpret and comprehend the outcomes. Due to this, putting investment strategies based on quantum computing techniques into practice may be difficult.
There are restrictions on the size and complexity of calculations that can be carried out with the available hardware because quantum hardware is still in its infancy. This may restrict the use of quantum computing in the financial sector.
Regulation and ethical issues: The application of quantum computing to the financial sector raises a number of regulatory and ethical issues, such as those involving data privacy, cybersecurity, and market fairness.
The advantages of quantum computing in finance could be significant despite these difficulties and restrictions. Quantum computing techniques are likely to be used more frequently in the financial sector as quantum hardware develops and becomes more widely available. The financial sector can unleash the full potential of quantum computing to boost investment performance, lower risk, and improve the security and integrity of financial markets by addressing these issues and constraints.